Video inpainting을 위한 soft split, soft composition
[arXiv]
[Github]
Abstract
Soft Split 및 Soft Composition 작업을 기반으로 하는 video inpainting 용 Transformer 모델인 FuseFormer 제안
Introduction
Vision Transformer(ViT) baseline +
- 여러 프레임에서 작동하도록 수정
- 고해상도 프레임들로 인한 계산 부담 완화를 위해 transformer block 전후에 가벼운 convolution 사용
Transformer의 패치 단위 연산으로 인해 sub-token level feature 간의 직접적인 상호작용이 부족하다.
따라서 이를 해결하기 위해 SS(Soft Split), SC(Soft Composition) 모듈 도입.
또한 transformer block을 해당 블록의 2계층 MLP를 각 MLP 사이에서 SS, SC를 수행하는 F3N(Fusion Feed Forward Network)로 대체하는 FuseFormer block으로 대체.
Method
ViB-T(Video inpainting Baseline with vanilla Transformer) + SS + SC = ViB-S
ViB-S + FuseFormer = ViF
Video inpainting Baseline with Transformer
ViB-T의 구성:
- Convolutional encoder, decoder
- Encoder, decoder 사이의 transformer blocks
- 위 둘 사이의 patch-to-token, token-to-patch module
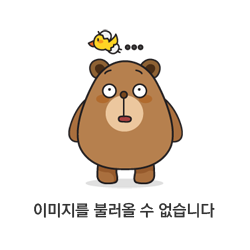
먼저 CNN으로 해상도를 줄인 후 모든 프레임의 모든 패치를 인코딩, transformer blocks를 지나고 다시 CNN을 통과하여 출력.
Soft Split (SS) and Soft Composite (SC)
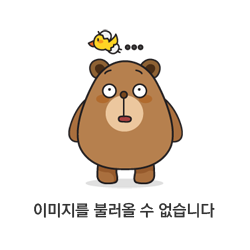
패치를 분할할 때, 이웃 패치끼리 겹치도록 분할하고(SS) 나중에 합칠 때는 겹치는 부분의 픽셀을 합산(SC).
FuseFormer
Transformer block을 대체할 FuseFormer block.
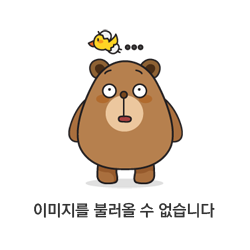
레이어 정규화(LN) 후 self-attention, 또 LN 후 F3N.
Fusion Feed Forward Network (F3N)
F3N의 공식화:
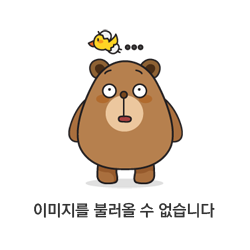
(각 프레임 t, 각 프레임 내의 각 토큰 n, fi = token vector)
간단하게 보면
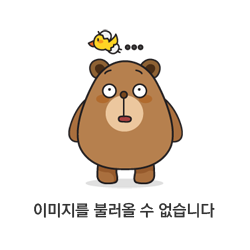
(다시 합치고 분할하면 안한 거랑 뭐가 다른 거야?라고 생각할 수 있지만 생각해 보면 겹치는 부분의 값이 달라짐을 알 수 있다.)
겹치는 부분에 대한 정규화 도입.
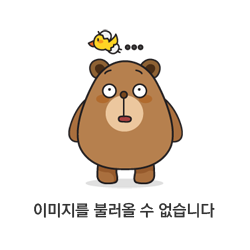
(1은 모든 값이 1인 행렬)
Training Objective
영상이 실제 영상인지 합성된 영상인지 구별하는 판별기 D를 도입.
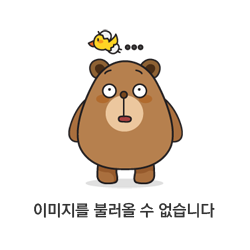
Total loss function : FuseFormer의 적대적 손실 + 간단한 재구성 손실
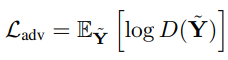
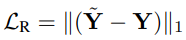

Experiments
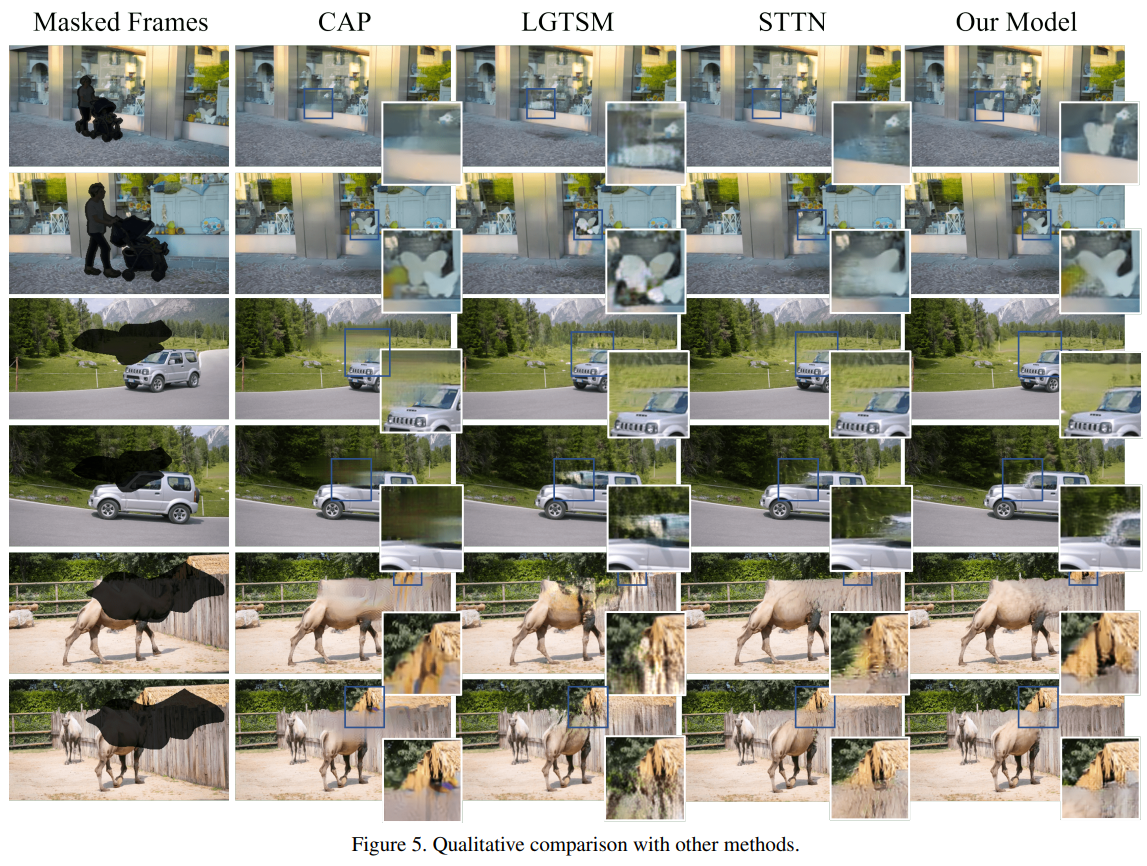
'논문 리뷰 > Vision Transformer' 카테고리의 다른 글
Semantic-SAM: Segment and Recognize Anything at Any Granularity (1) | 2023.10.18 |
---|---|
ProPainter: Improving Propagation and Transformer for Video Inpainting (3) | 2023.10.12 |
Flow-Guided Transformer for Video Inpainting (FGT) (0) | 2023.10.12 |
StyleDrop: Text-to-Image Generation in Any Style (1) | 2023.09.26 |
Fast Segment Anything (FastSAM) (0) | 2023.07.14 |
Self-Supervised Learning from Images with a Joint-Embedding Predictive Architecture (I-JEPA) (0) | 2023.07.08 |